Introduction
In the twelve years since I trained my first toy convolutional net on the CIFAR‑10 dataset, the ground has shifted under our feet. Transformers rewrote the playbook, GPUs became the new steam engines in AI advancements, and every industry I can name now treats “AI strategy” as table stakes rather than a moon‑shot. Yet amid the hype cycles and stock ticker headlines, the most interesting question remains stubbornly human: what is the future of AI, and how will it reshape how we learn, work, and understand ourselves?
what is the Future of AI at a glance
The Momentum We Can No Longer Ignore
- Transformers rewrote how models handle sequences and context.
- GPUs are the “steam engines” powering modern AI.
- AI strategy is baseline business practice across industries.
- Hype cycles often obscure deeper social implications.
- Past CIFAR‑10 breakthroughs feel quaint today.
- Automation headlines mask philosophical questions.
- We’re redefining work, learning, and human identity.
- Understanding AI’s path means understanding ourselves.
- Stock‑ticker news misses the human‑centric core.
- The true impact goes beyond mere efficiency gains.
Learning That Learns Back
- LLM‑powered Slack bots handle routine student queries.
- Automation frees instructors to tackle curiosity and doubt.
- Emotional telemetry senses frustration in real time.
- Gaze and pause metrics can out‑predict traditional exams.
- Adaptive tutors preempt learning roadblocks.
- Hyper‑personalization risks removing productive struggle.
- Serendipitous failures spark deep “aha” moments.
- Design must balance guidance with challenge.
- AI mentors must preserve human discovery sparks.
- Future classrooms merge data and empathy seamlessly.
Social Media’s Mirror Maze
- AI drives recommenders, moderation, and generative widgets.
- Next‑gen models decode slang before it hits Urban Dictionary.
- Entire micro‑communities can be synthesized on demand.
- Authenticity fatigue arises when real and fake blur.
- “Organic” vs. “lab‑grown” labels signal provenance.
- Diffusion models can create deceptive deepfakes.
- Watermarks and cryptographic tags guard authenticity.
- Media literacy must accompany technical defenses.
- Disinformation becomes an AI arms race.
- Trust hinges on transparency, not just tech fixes.
Machine Learning’s Engine Room
- Explainability by construction embeds causal structure.
- Interpretable architectures replace opaque black boxes.
- Federated learning keeps sensitive data local.
- Smartwatch updates train global health without leaks.
- Quantum‑classical hybrids hint at niche speed‑ups.
- Research shifts from scale to smarter model design.
- Data curation often beats brute‑force scale training.
- Smarter, not just bigger, defines next‑gen AI.
- Responsible AI demands both performance and clarity.
- Labs revisit fundamentals, not just hardware throttle.
Peering Toward 2050
- Autonomy everywhere—from drones to contract review.
- AGI labels aside, economic shifts will be seismic.
- Compute metered like utilities (petaflop‑hours as kWh).
- Geopolitics pivot around semiconductor supply chains.
- Society redefines “work” as rote tasks fade away.
- Shorter, portfolio‑based careers become normal.
- Craft revival thrives when automation handles grunt tasks.
- Human identity evolves alongside intelligent tools.
- Long‑term forecasting blends science and values.
- Our current choices shape the 2050 human‑AI partnership.
Marketing, Commerce & the Quantified Consumer
- Real‑time bidding auctions attention in under 200 ms.
- Mood‑adaptive creatives detect user anxiety.
- 3D avatars render outfits to your physique & schedule.
- Swarm pricing agents negotiate costs every minute.
- Hyper‑personalization risks slipping into manipulation.
- Consent dashboards must be as visible as “Buy Now.”
- Transparency toggles combat hidden data nudges.
- Concierge‑grade recommendations raise expectations.
- Ethical marketing demands clear opt‑outs.
- Respectful AI commerce builds consumer trust.
Robots Learn to Read the Room
- Multimodal robots combine LiDAR, vision, audio, LLMs.
- Emergent lines (“B or C?”) show safety fine‑tuning.
- Physical dexterity meets social intelligence.
- Collaborative arms predict human body language.
- Diffusion policy RL accelerates real‑world skills.
- Formal verification mirrors aerospace standards.
- Black‑box recorders log robot decision tracks.
- Ethical deployment requires human‑in‑loop checks.
- Trust builds via predictable, transparent behaviors.
- Factories become safe human‑robot hybrids.
Chatbots Grow a Heart—and a Spine
- LLMs surprised creators with emergent reasoning.
- Affect‑aware dialogue mirrors user tone and pacing.
- Sentiment analysis and prosody detection power empathy.
- Mental‑health pilots show higher self‑disclosure rates.
- Psycholinguistics guides emotionally intelligent responses.
- Radical transparency: bots admit they aren’t human.
- Utility over feigned emotion builds user trust.
- Clear disclaimers prevent misplaced reliance.
- Designs balance helpfulness with honest lack of feeling.
- Authenticity trumps imitation in chatbot UX.
Agents That Act, Not Just Chat
- Auto‑GPT, BabyAGI, Adept, Manus: goal‑driven autonomy.
- High‑level objectives spawn task decomposition loops.
- Agents call APIs, navigate GUIs, even write code.
- They observe outcomes, refine plans, repeat.
- Debugging agents feels like live algorithmic tuning.
- Reliability, not raw power, limits adoption today.
- “Agent supervisors” track hallucinations and budgets.
- Tool orchestration resembles Kubernetes for AI tasks.
- White‑collar roles will partner with digital assistants.
- Future workflows blend human oversight with agent action.
Health‑Care’s Administrative Vein
- AI automates ICD‑10 coding with high precision.
- Ambient transcription feeds real‑time medical coding.
- HL7/FHIR integration is critical for seamless EHRs.
- Edge‑case compliance still requires human review.
- Clinician workload shifts from paperwork to patient care.
- Data privacy safeguards must protect sensitive records.
- Automated audits reduce billing errors and denials.
- Population health analytics update on the fly.
- Interoperability drives faster, more accurate claims.
- Human oversight remains essential for complex cases.
Ethics Is Not an Appendix
- Measure demographic parity, robustness, and energy cost.
- Build contestability: let users appeal AI decisions.
- Keep a human circuit‑breaker in high‑stakes domains.
- Share AI dividends via retraining and social services.
- Privileged data must never be memorized or exposed.
- Bias mitigation requires ongoing audits and tests.
- Accountability frameworks assign clear responsibility.
- Transparency in models fosters public trust.
- Social safety nets smooth workforce transitions.
- Sustainable AI governance demands multi‑stakeholder input.
Regulation: The Art of Slow Enough
- Lawmakers lag behind rapid AI research advances.
- EU AI Act, CCPA, China’s registry show varied approaches.
- Adaptive governance with sunset clauses is vital.
- Transparency requirements help researchers innovate safely.
- Global harmonization avoids conflicting mandates.
- Draconian rules risk pushing models into the shadows.
- Sector‑specific guidelines balance risk and innovation.
- Public input and iterative rule‑making improve outcomes.
- Regulators must maintain open dialogues with labs.
- Flexible frameworks outpace rigid, one‑off legislation.
What 2025 Might Actually Feel Like
- Email clients auto‑draft replies but humans tweak tone.
- Agent clusters crunch market data overnight for insights.
- Adaptive tutors highlight gaps, then notify teachers.
- Logistics robots share elevators with polite floor announcements.
- Voice assistants translate cultural jokes, not literal text.
- Generative AI becomes a standard content tool in apps.
- AR/VR paired with AI for immersive training experiences.
- Edge computing powers low‑latency personal agents.
- No‑code AI platforms let non‑experts build simple agents.
- Ethics education enters school curricula alongside coding.
Global Reverberations
- McKinsey projects trillions in annual AI value.
- OECD warns of double‑digit percentage job displacement.
- Distribution curves tell local impact stories, not just totals.
- Rural clinics use ultrasound AI to save lives.
- Chatbots displace call‑center workers, demanding reskilling.
- Machine learning trims megawatts from data‑center cooling.
- Material‑discovery models hunt better battery chemistries.
- AI can ally with climate efforts if governance aligns incentives.
- Equitable deployment prevents new digital divides.
- Global collaboration is key for shared AI prosperity.
Table of Contents
1. What Is the Future of AI in Education?
When I taught an introductory deep learning class last spring, my teaching assistant was an LLM running inside a Slack bot—an early glimpse of what is the future of AI in personalized education.. It handled routine questions—“Why is my loss NaN?”—with eerie calm, freeing me to spend office hours on the messy stuff: confidence, curiosity, impostor syndrome. That, in miniature, is where what is the future of AI in education shines—automating the drudgery so that teachers can do what only humans can.
The next leap in exploring what is the future of AI is emotional telemetry. Consider a system that senses a student’s micro‑expressions, notices frustration rising, and quietly suggests a gentler explanation before the pupil gives up. A prototype already logs gaze patterns and pause lengths to predict comprehension better than the midterm exam (Adaptive learning). Give it five years and your algebra workbook will feel like a mentor who knows when you’re bluffing.
But personalization is a two‑edged sword. If every learner follows an algorithmic path tuned to prior proficiency, what happens to the serendipity of wrestling with ideas slightly out of reach? My own growth is littered with glorious failures—a physics proof I botched for weeks before the revelation struck in the shower. We must design systems that leave room for productive struggle, not just frictionless success.
2. What Is the Future of AI in Social Media?
Scroll any major platform today and you’re already conversing with neural nets—recommenders, moderation filters, generative text widgets—a taste of what is the future of AI in social media. The next generation goes deeper: models that decode slang before it hits Urban Dictionary, that detect nascent harassment without silencing reclaiming speech, and that synthesise entire micro‑communities on demand (social media AI).
And yes, the disinformation arms race—central to what is the future of AI—is very real. When a timeline contains equal parts human heartbreak and beautifully crafted synthetic sentiment, our empathy circuit may simply throttle down. Designers will need to signal provenance—think “organic” and “lab‑grown” labels for content—so that users can choose their diet intentionally rather than swallowing a homogenous puree (content moderation).
And yes, the disinformation arms race is real. The same diffusion model that paints your vacation selfie in Van Gogh hues can splice a political opponent’s face into a crime‑scene still. Technical countermeasures (watermarks, cryptographic provenance) help, but the deeper fix is cultural: teaching media literacy as early and rigorously as we teach long division.
3. Machine Learning’s Engine Room: What Is the Future of AI and Machine Learning
Beneath the user‑facing fireworks, in the search for what is the future of AI, labs are revisiting fundamentals.
- Explainability by construction. A new wave of interpretable architectures embeds causal structure from the outset, not as a retrofitted afterthought (XAI).
- Federated everything. In pondering what is the future of AI, as privacy regs tighten, models commute to data rather than the reverse. Your smartwatch updates a global ECG model overnight, never revealing raw heartbeats.
- Quantum flirtations. Noisy qubits can barely keep a thought, but hybrid workflows (classical pre‑processing, quantum kernels, classical post‑training) hint at exponential speed‑ups for niche problems. File it under “monitor, don’t hype—yet.”
The takeaway: progress in core algorithms is no longer synonymous with bigger GPUs. Architecture search, theoretical grounding, and clever data curation matter as much as teraflops.
4. What Is the Future of AI by 2050?
Forecasting 25 years out is closer to fiction than science, so let me stake three tentative claims:
- Autonomy everywhere. Understanding what is the future of AI, from cargo drones to legal contract review, systems will act without human micro management. Whether you call it AGI or not, the economic shift will be seismic.
- Compute as infrastructure. By mid‑century, petaflop‑hours will be metered like electricity. Nations will argue over semiconductors as they now battle for oil fields.
- New human identities. When AI handles the rote, society may re‑negotiate what counts as “work.” Expect shorter professional cycles, portfolio careers, even a revival of craft for craft’s sake.
5. What Is the Future of AI in Digital Marketing
Marketers have always wanted to read your mind; what is the future of AI just hands them a louder stethoscope. Real‑time bidding already auctions your attention in 200 ms. Next comes mood‑adaptive creative: the banner ad that senses anxiety (subtle facial tension, slower scrolls) and swaps its palette to soothing pastels (digital marketing).
On the retail side, recommendation engines graduate to concierge status—another glimpse of what is the future of AI. Imagine opening a fashion site and seeing a single outfit, rendered on a 3D avatar shaped exactly like you, tailored to today’s weather and your upcoming calendar appointments.
The efficiency gains are intoxicating, but when pondering what is the future of AI, personalization can slip into manipulation. When a system knows you better than you know yourself, consent becomes slippery. Transparency dashboards and opt‑out toggles must be as prominent as “Buy Now” buttons, not buried in legal sludge.
6. What Is the Future of AI and Robotics
My lab recently tested a warehouse robot armed with multimodal perception—LiDAR, vision, audio, and an LLM for high‑level planning. During a fire‑drill it halted, turned to the nearest human, and asked, “Evacuation procedure B or C?” No one had programmed that line; it emerged from a safety‑policy fine‑tune (robotics).
The frontier in defining what is the future of AI is this blend of physical dexterity and social intelligence. Collaborative arms must predict not only joint torques but also human intentions: is the worker about to pivot left? That sigh—frustration or fatigue? Safety certification for autonomous systems will look like aerospace: redundant sensors, formal verification, black‑box “flight recorders.”
7. Chatbots Grow a Heart—and a Spine: What Is the Future of AI Chatbots
Large language models surprised even their creators with emergent reasoning, but conversation alone is table stakes now. The cutting edge is affect‑aware dialogue. Pilot studies show that when a mental‑health chatbot mirrors your syntactic rhythm—short sentences when you’re terse, gentle hedges when you’re uncertain—self‑disclosure increases measurably (AI chatbots).
The technical recipe blends sentiment analysis, prosody detection, and a dash of psycholinguistics. The deeper question: should a bot feign empathy it does not feel? I lean toward radical transparency: let users know the system is synthetic, yet capable of genuine utility if not genuine emotion. Authenticity trumps imitation.
8. Agents That Act, Not Just Chat: The Automated Horizon for Autonomous AI Agents
Auto‑GPT, BabyAGI, Adept, Manus—names piling up like new elements on the periodic table. What unites them is their shift from text completion to goal‑directed autonomy. Give the agent a high‑level objective (“Compare the top five cyber‑insurance plans for a 50‑person startup and draft a recommendation”) and it will spawn mini‑tasks, call APIs, navigate GUIs, even write Python, all while critiquing its own performance (autonomous agents).
Watching them feels like debugging in real time: you see them loop, correct, overfit, recover. They are brilliant one moment, baffled the next. Reliability, not raw capability, is the bottleneck. Expect a cottage industry of “agent supervisors” to track hallucination risk, budget spend, and safety constraints—much like Kubernetes orchestrates containers today.
9. Health‑Care’s Administrative Vein: What Is the Future of AI in Medical Coding
Few dream of becoming a medical coder; fewer still enjoy deciphering ICD‑10 minutiae. Here AI’s value proposition is mercifully clear: handle the paperwork so clinicians can practice medicine. Early systems flag mismatched procedure codes with impressive precision (medical coding).
Long term, envision a seamless loop: a patient encounter feeds an ambient transcription model; entities map to standardized codes; billing, auditing, and population‑health analytics update in near real time. Humans remain in the loop for edge cases and compliance—freeing cognitive energy for attentive bedside care.
10 Ethics Is Not an Appendix: Ethical Implications of AI
Every section above raises moral questions that cannot be patched after the fact. Bias creeps in through skewed data; privacy evaporates when models memorize sensitive chunks; labor markets lurch as tasks evaporate. I have no silver bullet, but four guidelines anchor my practice:
- Measure first. If you don’t quantify demographic parity, robustness, energy cost—you’re guessing.
- Build for contestability. Build for contestability. As what is the future of AI unfolds, users should appeal a model decision and get a usable explanation, not a probability score.
- Keep a human circuit‑breaker. Especially in high‑stakes domains. Automation bias is real; fail‑safe design matters.
- Share the upside. AI’s dividends ought to fund retraining, universal basic services, or whatever social contract we negotiate collectively.
11. Regulation: The Art of Slow Enough
I once joked that lawmakers operate at 96 baud while AI research streams at 10 gigabit—yet probing what is the future of AI demands regulators catch up. But the gap is closing. The EU’s AI Act, California’s data laws, China’s algorithm registry—all imperfect, all necessary. My plea to regulators: embrace adaptive governance. Draft principles, require transparency, demand sunset clauses, and iterate. The worst fate is paralysis: draconian rules that stifle open research while leaving black‑box models in the shadows (EU AI Act).
12 What 2025 Might Actually Feel Like: AI Advancements 2025
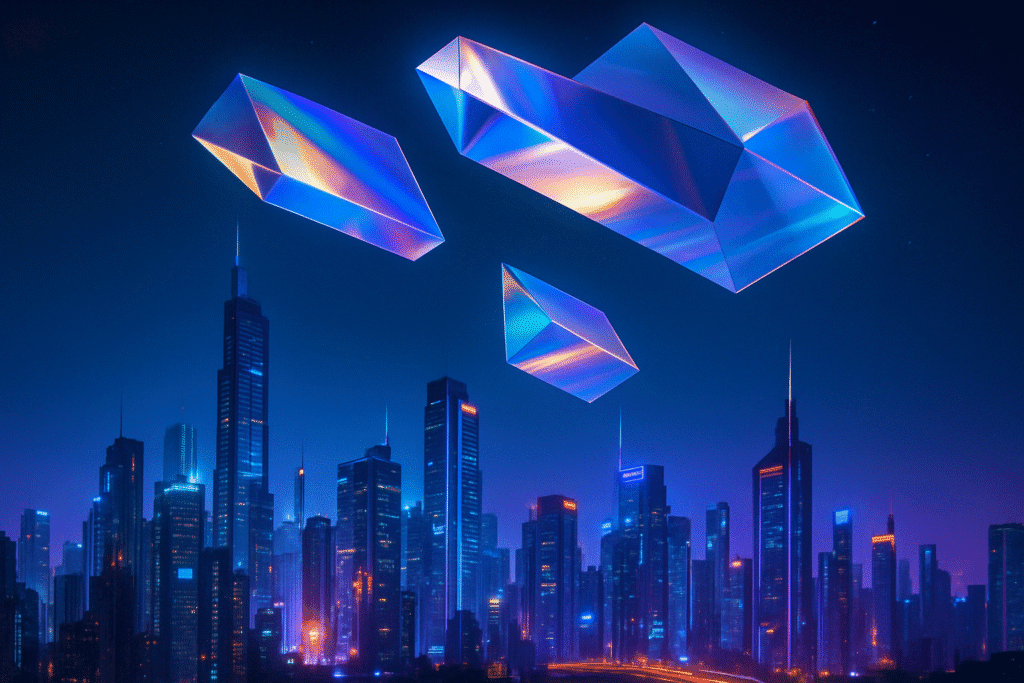
Near‑term forecasts are safer, so let me paint a plausible Tuesday two years hence—an era of ai advancements 2025:
- Your email client auto writes replies—a snapshot of what is the future of AI in everyday communication—but you edit the important ones because tone still matters.
- At work, an agent cluster crunches market data overnight and suggests pivot options before the daily stand‑up.
- Your teenager’s homework is graded by an adaptive tutor—a clear example of what is the future of AI in education—that highlights—not fixes—conceptual gaps, then notifies the human teacher.
- A logistics robot shares the elevator with you, politely announcing its floor, a small courtesy mandated by building code.
- Out of habit, you ask your voice assistant to translate a meme into Urdu; it annotates cultural jokes rather than literal words.
13. Global Reverberations
The macro economics are staggering—McKinsey pegs potential annual AI value at trillions of dollars; the OECD warns of double digit job displacement—forcing us to reckon with what is the future of AI at scale. Personally, I find the distribution curves more instructive than the aggregate sum. A rural clinic with an ultrasound AI can save lives; a call‑center worker replaced by a chatbot needs new skills—and rent money—in sixty days. We must track both stories with equal rigor.
On climate, machine‑learning optimizers already trim megawatts from data‑center cooling. Pair that with material‑discovery models searching for better batteries and AI becomes a climate ally rather than villain. The catch, again, is governance: aligning research incentives with planetary well‑being, not quarterly profit.
Closing Reflections
When I re‑read Turing’s 1950 paper, I’m struck by how little he cared about hardware. His genius was recognizing the substrate‑independent nature of thought. Today we face the inverse challenge: minds of silicon are blooming faster than our social scaffolding can adapt.
I remain, at heart, a techno‑optimist. The same creativity that birthed these systems can guide their stewardship—if we keep the conversation honest. In practical terms that means building transparent models, auditing bias relentlessly, championing open education, and designing jobs that amplify human judgment rather than sideline it.
The tea with Turing and Wiener is almost finished. Before they fade from my imaginary kitchen, I ask a final question: “Will we get this right?” Turing smiles cryptically; Wiener raises an eyebrow. The answer, as always, is up to us.
A Note to the Reader
Everything in this essay reflects my own observations, experiments, and late night debates with colleagues as we wrestle with what is the future of AI. I welcome disagreement—civil, data‑driven, and curious. After all, the future of AI is not pre‑written code; it’s a collaborative commit waiting for our pull request.